In today’s digital world, businesses need more than just traditional marketing to attract and retain…
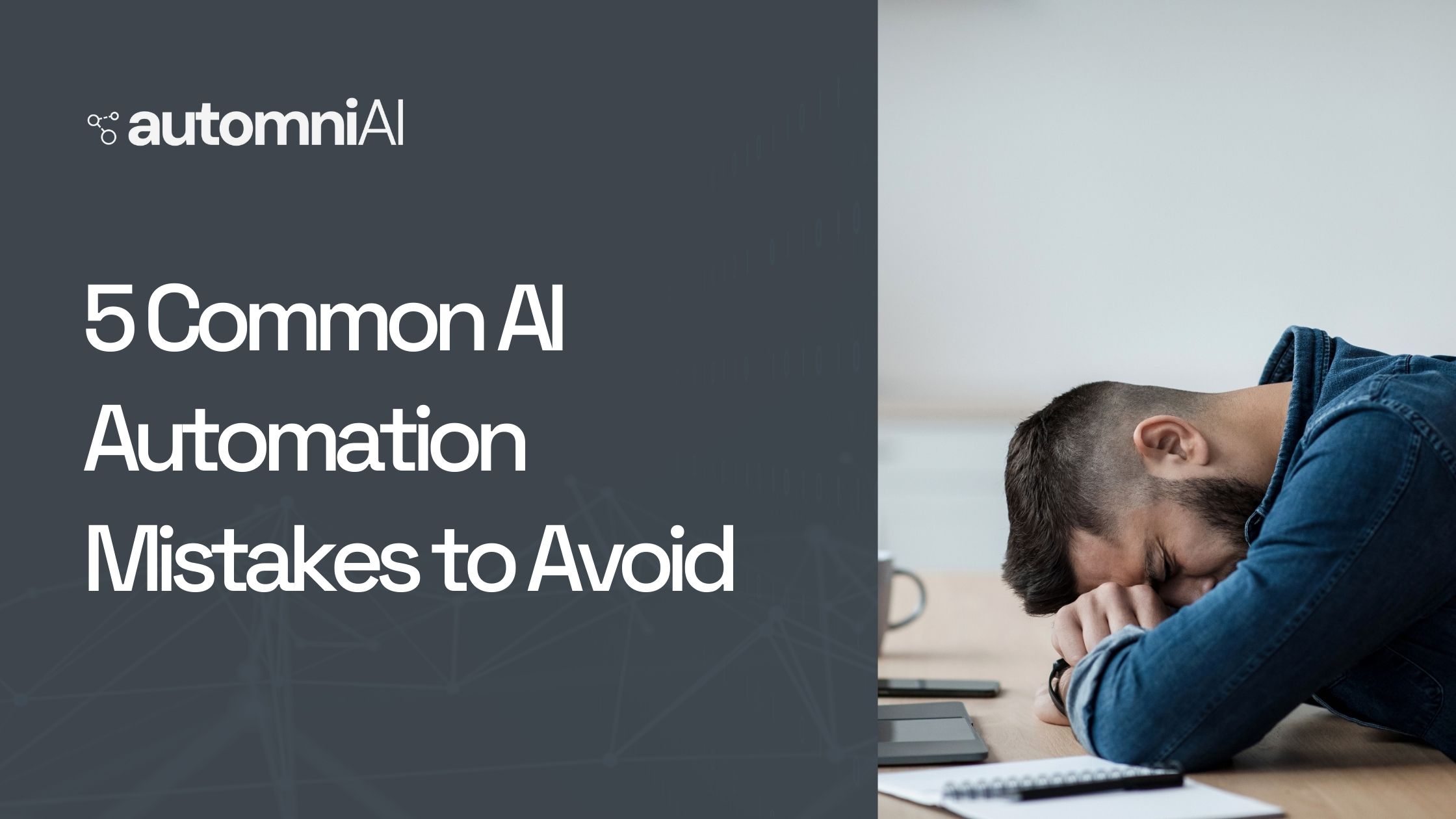
5 Common AI Automation Mistakes to Avoid
Introduction
AI automation has revolutionized industries, streamlining operations and improving efficiency. However, despite its potential, many businesses struggle with common pitfalls that hinder success. Whether due to unclear goals, poor data management, or over-reliance on AI, these mistakes can lead to inefficiencies and costly errors.
To ensure a smooth AI automation journey, it’s crucial to recognize and avoid these pitfalls. This article explores five common AI automation mistakes and how to prevent them.
1. Lack of Clear Objectives
One of the biggest mistakes businesses make when implementing AI automation is failing to establish clear objectives. Without well-defined goals, AI initiatives often lack direction, resulting in wasted resources and suboptimal outcomes.
1.1. Understanding Business Needs
Before adopting AI automation, businesses must align their strategies with organizational needs. Implementing AI without a clear purpose leads to inefficiencies. Common mistakes include:
- Deploying AI tools without knowing how they fit into existing workflows
- Automating tasks that don’t require AI, leading to unnecessary expenses
- Failing to define success metrics, making it difficult to measure effectiveness
To avoid this, businesses should start by identifying specific problems AI can solve. For example, if customer service response time is an issue, AI chatbots could be a valuable solution.
1.2. Setting Measurable KPIs
Without measurable KPIs (Key Performance Indicators), businesses cannot accurately assess AI’s impact. Some useful KPIs include:
- Response time improvements in customer service
- Reduction in manual workload
- Cost savings from automation
Using AI analytics tools, such as Google Cloud AI or IBM Watson, can help track these metrics and ensure automation meets business goals.
2. Ignoring Data Quality
AI relies heavily on data, and poor data quality can lead to inaccurate predictions, biased decisions, and automation failures.
2.1. Ensuring Data Accuracy and Consistency
Many businesses make the mistake of feeding AI systems with outdated, incomplete, or inconsistent data. Common issues include:
- Duplicate records that lead to incorrect insights
- Inconsistent data formats across different sources
- Lack of real-time updates, resulting in outdated AI decisions
To improve data quality, businesses should:
- Regularly clean and preprocess data
- Use structured databases for better organization
- Automate data validation processes
2.2. Avoiding Bias in AI Models
Bias in AI can lead to unfair and unethical outcomes. If AI models are trained on biased data, they may reinforce discrimination in hiring, lending, and law enforcement. To mitigate bias:
- Use diverse and representative datasets
- Conduct regular bias audits on AI models
- Implement fairness-enhancing AI tools like IBM AI Fairness 360
3. Over-Automating Processes
While automation can improve efficiency, over-reliance on AI without human oversight can backfire.
3.1. Recognizing Tasks That Require Human Oversight
AI should enhance human capabilities, not replace them entirely. Some tasks require critical thinking and human judgment, such as:
- Legal decision-making
- Customer service in complex situations
- Medical diagnoses requiring personalized attention
Companies like Amazon and Facebook have faced issues with over-automation, such as AI-driven hiring biases and misinformation filtering failures.
3.2. Implementing AI-Human Collaboration
Successful AI integration involves a balance between automation and human expertise. For example:
- AI chatbots handle basic customer queries, while human agents manage complex issues
- AI-powered data analysis provides insights, but humans make final decisions
By maintaining this balance, businesses can prevent AI from making costly errors while still benefiting from automation.
ChatGPT said:
4. Neglecting AI Maintenance and Monitoring
Implementing AI automation isn’t a one-time task. AI models require regular updates, monitoring, and fine-tuning to ensure they perform efficiently. Businesses that neglect AI maintenance risk outdated, inaccurate, and inefficient automation.
4.1. Continuous Training and Model Updates
AI learns from data, but data evolves. If AI models aren’t continuously updated, they may become less accurate over time. Common issues include:
- AI chatbots failing to understand new slang or customer queries
- Predictive analytics tools providing outdated recommendations
- Fraud detection systems missing new types of fraudulent activities
To avoid these problems, businesses should:
- Regularly retrain AI models with fresh data
- Implement machine learning pipelines that allow automated updates
- Use AI platforms that support adaptive learning, such as TensorFlow and PyTorch
4.2. Monitoring AI Performance Metrics
AI systems must be continuously evaluated to ensure they align with business goals. Without proper monitoring, AI can drift from its intended purpose. Key AI performance metrics include:
- Accuracy: How often the AI provides correct predictions
- Response Time: The speed at which AI processes information
- Error Rate: Frequency of incorrect outputs
- User Satisfaction: Feedback from customers and employees using AI
Companies can use AI monitoring tools like Microsoft Azure AI and Google AI Platform to track performance and detect inefficiencies early.
5. Underestimating Ethical and Compliance Issues
AI automation comes with ethical and legal responsibilities. Many businesses fail to consider privacy concerns, bias, and compliance regulations when deploying AI systems, leading to potential legal troubles and reputational damage.
5.1. Understanding AI Regulations
Laws surrounding AI are evolving rapidly, and businesses must stay compliant. Some key regulations include:
- GDPR (General Data Protection Regulation): Governs data privacy in Europe
- CCPA (California Consumer Privacy Act): Protects consumer data in the U.S.
- AI Act (EU): Proposed regulation for ensuring safe and transparent AI
Failing to adhere to these laws can lead to hefty fines and lawsuits. To ensure compliance, businesses should:
- Regularly audit AI-driven processes
- Implement strong data privacy measures
- Seek legal consultation on AI policies
5.2. Ensuring Transparency in AI Decisions
Many AI systems operate as “black boxes,” making it difficult to understand how decisions are made. This lack of transparency can create trust issues among users and regulators.
To promote AI transparency:
- Use explainable AI (XAI) methods to interpret AI decisions
- Provide users with clear explanations of AI-driven outcomes
- Document AI decision-making processes for accountability
Companies like IBM and Google are leading efforts to make AI more explainable, offering tools like IBM Watson OpenScale and Google’s Explainable AI platform.
Conclusion
AI automation offers immense benefits, but businesses must navigate potential pitfalls carefully. By avoiding common mistakes such as unclear objectives, poor data quality, over-automation, lack of maintenance, and ethical neglect, companies can maximize the value of AI.
To ensure successful AI implementation:
- Define clear business goals before automating processes
- Maintain high-quality, unbiased data for AI training
- Balance automation with human expertise
- Continuously monitor and update AI models
- Stay compliant with AI regulations and ethical standards
By taking these precautions, businesses can leverage AI to its full potential while minimizing risks.
FAQs
1. What is the biggest mistake businesses make when automating with AI?
The biggest mistake is implementing AI without clear objectives. Without a defined purpose, AI systems can become inefficient and fail to deliver the expected value.
2. How can I ensure my AI automation aligns with my business goals?
Start by identifying specific problems that AI can solve. Define measurable KPIs and track AI performance regularly to ensure alignment with business objectives.
3. What tools can help monitor AI performance effectively?
AI monitoring tools such as Microsoft Azure AI, Google AI Platform, and IBM Watson provide insights into AI performance, accuracy, and efficiency.
4. How do I prevent AI bias in my automation process?
To reduce AI bias, use diverse datasets, conduct regular bias audits, and implement fairness-enhancing AI tools like IBM AI Fairness 360.
5. Why is it important to have human oversight in AI automation?
AI lacks human intuition and ethical reasoning. Certain decisions, such as legal judgments or medical diagnoses, require human expertise to ensure fairness and accuracy.
Comments (0)